Self-Efficacy is a Stronger Predictor of Final Grade Than Motivation in an Introductory Biology Course: A Structural Equation Analysis
Abstract
Student self-efficacy, their confidence to successfully perform a task, is positively related to course performance. Students with high self-efficacy for a task are more motivated toward that task. Yet few studies have measured students’ self-efficacy and motivation levels after exams are returned in introductory biology classes or studied how these levels relate to final course grades. To address these questions, we used structural equation modeling to examine how introductory biology students’ (n = 123) self-efficacy and motivation scores at the beginning of the semester and after the first two course assessments related to their final course grades. Both self-efficacy and intrinsic goal orientation—motivated by a desire to learn or gain new skills—decreased after the first assessment grades were returned to the students. We found positive relationships between self-efficacy and motivation, and between self-efficacy and final course grades, across all timepoints. Motivation scores were not associated with final course grades, but the relationship between self-efficacy levels and final grades was significant and became stronger at each timepoint. These results suggest the importance of supporting self-efficacy development after early assessments, a time when students are particularly vulnerable to declines in confidence and motivation.
INTRODUCTION
Increasing undergraduate students’ self-efficacy (SE) has been identified as one way to help students perform well in their courses and persist in their degree programs (Schunk, 1984; Bandura, 1986; Pajares, 1996; Andrew, 1998; Komarraju and Nadler, 2013). SE refers to a person's belief that they can succeed at a specific task, and it is positively related to motivation for that task (Bandura, 1986). Motivation refers to which factors drive a student to succeed (Pintrich, 2000a, 2000b). Therefore, one goal would be for all students to have high or increasing levels of SE and motivation as they engage in a class. Yet, how SE and motivation levels change over a course and how those changes may impact course performance outcomes is less understood.
SE levels can fluctuate as students receive feedback on tasks (Ouweneel, et al., 2013; Beatson et al., 2018). For undergraduate students, SE levels in a class may be based on tasks such as earning a good grade on an assignment or learning the material in the course. For example, a positive outcome on an assessment such as an exam can be seen as evidence of success, increasing motivation and confidence to do well on future exams. Most biology courses have several summative assessments (e.g., exams or quizzes) throughout the semester, often comprising a large percentage of the points that contribute to student final grades. Given this, we posit that grades on summative assessments may provide one of the most salient feedback measures to inform SE in a course, providing tangible evidence of success or failure to the student. Such feedback may impact students’ motivation for future exams, as well as their SE levels. However, it is unclear how the relationships between assessment feedback, SE level, and motivation level may change over time, and how those may relate to final course grades. A better understanding of these relationships may help uncover critical feedback timepoints for students to build SE.
Changes in SE Over Time
SE beliefs about a novel task are thought to be malleable initially, but become more stable over time as individuals accumulate information about their performance on the task (Bandura, 1997; Lent et al., 1986; Zimmerman et al., 1992). In one study, researchers measured students’ SE at three timepoints over two semesters of introductory biology. They found evidence that students’ SE levels for biology achievement increased over the two semesters (Cole and Beck, 2022). Similarly, Ainscough and colleagues (2016) found that students in a first-year biology course had higher SE levels by the end of the semester-long course. These studies collectively suggest that SE is malleable for introductory biology students and typically increases over time. However, changes in SE levels are relative to when the initial measures are taken and neither of these studies measured SE levels before the course began, which may be a key baseline measurement. They also did not measure SE as students were receiving task feedback, a timepoint theoretically linked to adjustments in confidence levels. The lack of these specific timepoints means our understanding of how SE changes over time in introductory biology courses may be incomplete. Therefore, this study measured precourse student SE levels and compared them with measures immediately after students received their first two summative assessment grades in a biology class.
SE and Assessment Feedback
Enactive mastery experiences are those where an individual succeeds at a task. They have been proposed as one of the most impactful ways to increase SE according to SE theory (Bandura, 1977; Britner and Pajares, 2006; Usher and Pajares, 2006; van Dinther et al., 2011). In an introductory biology course, enactive mastery experiences are likely to occur when students receive what they consider to be successful scores on exams, tests, and quizzes. This positive feedback on assessments should help increase SE levels, while not doing well on assessments (negative feedback) may decrease SE levels (Chan and Lam, 2010; Beattie et al., 2016). In a study examining how introductory biology students defined success, Von der Mehden and colleagues (2024) found that 82% of students defined success as performing well on assessments. Based on these findings, successful performance on an exam or quiz (positive feedback) is likely to have a positive impact on students’ SE beliefs. Yet, there has not been a study assessing student SE levels immediately after exam feedback in introductory biology.
Goal Orientation Theory and SE
Goal orientation theory describes how students are motivated for tasks through two primary motivational orientations: performance and mastery (Pintrich 2000a, 2000b). For this study we use the term “extrinsic goal orientation” (EGO) to describe performance orientation and the term “intrinsic goal orientation” (IGO) to describe mastery orientation (Table 1). EGO is when students are motivated by achieving an external reward or validation (e.g., grades). Individuals motivated by an EGO may want to appear competent compared with those around them. Students motivated by an IGO have goals of learning for the sake of gaining new knowledge or skills. Studies suggest that students with IGOs, rather than EGOs, perform better in their courses (Mattern, 2005; Diseth, 2011), do better in the face of failure (Dweck and Legget, 1988; Niiya et al., 2004), and have higher SE levels (Geitz et al., 2016). Others have equated IGOs as intrinsic self-appointed standards, while EGOs are shaped by extrinsic norms (Pintrich and de Groot, 1990; Elliot and McGregor, 2001).
Constructs | Abbreviation | Brief Definition | Example |
---|---|---|---|
Self-efficacy | SE | Confidence to succeed at some task | A student who is confident that they will do well on an exam has high self-efficacy for that task. |
Intrinsic goal orientation | IGO | Motivated by internal rewards | A student is motivated to learn a new idea or skill. |
Extrinsic goal orientation | EGO | Motivated by external rewards or validation | A student is motivated to get a certain grade or appear smart. |
Just as student SE levels may change over time, motivation levels may also vary as students receive feedback about whether they met their standards for success or failure. Henderlong Corpus and colleagues (2020) studied undergraduate students’ motivational changes over their first year of college and found that students on average had high incoming IGO that decreased over time. They also found that on average students had initially low EGO that increased over the first year in college. Given the relationship between SE and motivation (Bandura, 1986), and the relationships between IGO and EGO to learning, it follows that there should be positive relationships between SE, motivation, and academic performance in a biology class.
Hypothesized Relationships in an Introductory Biology Class
Summative assessments in an introductory biology class often comprise a large part of a student's final grade. Therefore, measuring students’ SE, IGO, and EGO after exam grades are returned could be important given that summative assessment grades should theoretically be used as feedback for positive or negative changes in each of these constructs. We predict that SE will be positively related to both IGO and EGO, and that both IGO and EGO may mediate a positive relationship between SE and final course grades (Figure 1). We expect that relationships between SE, IGO, EGO, and final course grade will change over time from the initial pre-course baseline through the receipt of grades (feedback) on summative assessments. Prior work has found that students with higher SE levels tend to have higher IGO (Geitz et al., 2016) and other studies have shown that high IGO is related to high course performance (Mattern, 2005). We expect that the relationship between SE and IGO will be positively related to final course grades. SE levels alone are positively related to course grades (Chemers et al., 2001; Britner and Pajares, 2006; Hsieh et al., 2007), so we also expect the relationship between SE level and final grade to be positive. EGO is more complicated because studies have found varying relationships between EGO and course performance (Wolters et al., 1996; Middleton and Midgley 1997; Pajares et al., 2000). Researchers have speculated that these findings stem from differences in the two types of EGO: approach and avoidance (Elliot and Harackiewicz, 1996; Church et al., 2001). Extrinsic approach is when a student wants to earn a certain grade to appear competent, while extrinsic avoidance is when a student wants to avoid a low grade that would make them appear less competent (Pintrich, 2000a). Extrinsic avoidance has been shown to be detrimental to performance (Dweck and Leggett, 1988; Ames, 1992) while an extrinsic approach can be beneficial for students (Harackiewicz et al., 1997; Harackiewicz et al., 2000; Church et al., 2001). Given the mixed effect of EGO on students’ performance we are unsure if EGO will have a significant relationship with final grades.
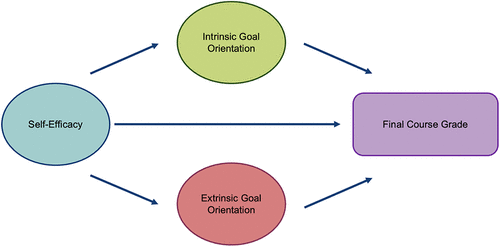
FIGURE 1. Diagram of relationships predicted by SE theory and goal orientation theory that will be tested in this study.
Study Rationale
The present study builds on SE and goal orientation theories to describe the relationships between SE, IGO, EGO, and final grades in introductory biology (Figure 1). While studies have found that student SE levels can shift in response to performance on tasks (Lent et al., 1986; Zimmerman et al., 1992; Bandura, 1997), few have examined how SE, IGO, and EGO levels vary and are related to each other across a semester. Previous research has also overlooked critical timepoints such as precourse and postassessment intervals, which theory suggests could be critical moments for influencing SE. Our study addresses these gaps by assessing SE, IGO, and EGO levels precourse as a baseline and then reassessing each postassessment 1 and postassessment 2. We then conducted analyses to address the following research questions:
How do SE, IGO, and EGO change after assessment grades are returned in introductory biology?
How do the relationships between SE, motivation, and final grades change after assessment grades are returned in introductory biology?
By examining how the relationships between SE, IGO, and EGO change over time in response to feedback on assessments, we aim to uncover timepoints that could be strategically leveraged by instructors to strengthen students’ SE throughout the semester. In turn, this could drive increased motivation and better academic outcomes, ultimately enhancing student success.
MATERIALS AND METHODS
Courses and Participants
The participants for this study were enrolled in four large lecture sections of introductory organismal biology at a research-intensive institution in the southeastern United States. The course is one of the requirements for the biology major and is typically the first biology course students take. Most students in the course are in their first or second year of college. Other science majors also take the course as a requirement of their major. The lecture sections had 156, 208, 156, and 234 students enrolled, respectively (754 total students), with the first two sections taught by the same instructor. This study was approved under Institutional Review Board protocol #23-07663-XP.
The lecture sections each had different assessment structures (Table 2). Lecturer A with two sections (156, 208) had seven, 65-point quizzes, and one final exam. Lecturer A specifically called their summative assessments “quizzes” in an effort to make them less intimating for students. The quiz with the lowest score was dropped and they offered exam wrappers (i.e., a postassessment assignment where students could earn points for correcting missed questions; students could earn two points for correcting any incorrect questions in these sections). Lecturer B with one section (234) had three 100-point exams and one final exam. No exam scores were dropped, and exam wrappers were only available for one or two of the most missed short-answer questions. Lecturer C with one section (156) had four 90-point exams and one final exam. None of the exam scores were dropped and there were no exam wrappers. All instructors had self-authored assessments with a mix of multiple-choice (∼70%) and short-answer (∼30%) questions. The final course grade across all sections was composed of 45–54% summative assessment, 26–35% formative assessment, and 20% discussion (a separate session run by graduate students). Courses are coordinated across instructors so that content and point distributions are relatively similar.
Lecturer A | Lecturer B | Lecturer C | ||
---|---|---|---|---|
Section | 1 | 2 | 3 | 4 |
Summative assessments | Seven 65 pt quizzes and one final exam | Seven 65 pt quizzes and one final exam | Three 100 pt exams and one final exam | Four 90 pt exams and one final exam |
Drops | Lowest quiz dropped | Lowest quiz dropped | No | No |
Exam-wrappers | Yes | Yes | Yes | No |
Points available from Final Exam | 150 pts | 150 pts | 150 pts | 90 pts |
Points available from summative assessments | 540 pts | 540 pts | 450 pts | 450 pts |
Total points available in the course | 1000 pts | 1000 pts | 1000 pts | 1000 pts |
Data Collection
Data collection occurred during the fall semester of 2023. Three surveys were distributed by instructors to students via email (Supplemental 1). Student participation was incentivized with one extra credit point per survey. The first survey (precourse) was distributed before the semester started and closed before the first day of class to collect data on SE, IGO, and EGO before interacting with the instructor (open Aug. 19–23). The second survey (postassessment 1) was distributed after students received their grades from their first assessment and was open for 1 week; this timing depended on the class (Sept. 7–14; Sept. 21–28; Oct. 4–12). The third survey (postassessment 2) was distributed after students received their grades for the second assessment, and again, the distribution dates were adjusted by class (Sept. 24–Oct.1; Oct. 19–26; Nov. 1–8). We worked closely with each instructor to monitor when the assessment grades were posted and available to students to time the distribution of the survey for each class. Assessment grades were available to students during the time they were surveyed.
Survey Instrument
The surveys were created by researchers using Qualtrics software. The survey included several subscales of the Motivated Strategies for Learning Questionnaire (MSLQ). The MSLQ is a commonly used survey instrument for measuring motivational constructs based on social cognitive theory (Pintrich et al., 1991). The MSLQ has 15 subscales to measure distinct motivation constructs, and each subscale can be used independently of the others. Three subscales of the MSLQ were chosen for this study (Table 3). They measured IGO, EGO, and SE using Likert scale items. For each item, students could select a rating from 1–7 where 1 was “not at all true of me” and 7 was “very true of me.” The subscale items were ordered based on the original MSLQ, meaning the items in each subscale were not grouped together, but were dispersed through the survey.
The IGO subscale had four items that asked about the degree to which a student enjoyed challenges in courses and learned/mastered the content in the course. For example, one of the items on the IGO subscale asked students to rank how aligned the following statement is to their perspective of the class “In a class like this, I prefer course material that really challenges me so I can learn new things.”
The EGO subscale had four items about the degree to which a student participated in a course for external rewards such as grades and how others perceived them. For example, one of the items on the EGO subscale asked students to rank how aligned the following statement is to their perspective of the class: “The most important thing for me right now is improving my overall grade point average, so my main concern in this class is getting a good grade.”
The SE subscale had eight items about students’ expectations of their performance and their confidence. For example, one of the items on the SE subscale asked students to rank how aligned the following statement is to their perspective of the class: “I am confident I can understand the basic concepts taught in this course.” The SE section was specifically focused on SE for learning and performance and did not include SE for other tasks.
With the consent of the students, final numeric course grades were collected from instructors at the end of the course and were matched to students’ responses using their school ID code. Student sociodemographic characteristics (gender, race and/or ethnicity, college generation, year in college, major, and lecture section) were collected from students to describe the student population included in this study.
Data Analysis
Participants.
Partial survey responses were removed from the dataset. Complete responses for the three surveys were then matched across timepoints to each student using their school ID code. Only students who responded to all three surveys were included in the final dataset (n = 123). While this greatly reduced the sample, it also allowed us to analyze shifts over time among the same sample of students. Participants were primarily women (78.9%), White (79.7%), continuing generation students (87.0%) in their first year of college (71.5%), in the biological sciences major (43.9%), and were taught by Lecturer A (Section 1 - 32.5%, Section 2 - 42.3%; Table 4).
Subscale Construct | Items |
---|---|
Intrinsic Goal Orientation | In a class like this, I prefer course material that really challenges me so I can learn new things. |
In a class like this, I prefer course material that arouses my curiosity, even if it is difficult to learn. | |
The most satisfying thing for me in this course is trying to understand the content as thoroughly as possible. | |
When I have the opportunity in this class, I choose course assignments that I can learn from even if they don't guarantee a good grade. | |
Extrinsic Goal Orientation | Getting a good grade in this class is the most satisfying thing for me right now. |
The most important thing for me right now is improving my overall grade point average, so my main concern in this class is getting a good grade. | |
If I can, I want to get better grades in this class than most of the other students. | |
I want to do well in this class because it is important to show my ability to my family, friends, employer, or others. | |
Self-Efficacy for Learning and Performance | I believe I will receive an excellent grade in this class. |
I'm certain I can understand the most difficult material presented in the readings for this course. | |
I'm confident I can understand the basic concepts taught in this course. | |
I'm confident I can understand the most complex material presented by the instructor in this course. | |
I'm confident I can do an excellent job on the assignments and tests in this course. | |
I expect to do well in this class. | |
I'm certain I can master the skills being taught in this class. | |
Considering the difficulty of this course, the teacher, and my skills, I think I will do well in this class. |
Sociodemographic Characteristics | % (n = 123) | |
---|---|---|
Gender | Woman | 78.9 |
Man | 21.1 | |
Race and/or Ethnicity | Asian | 5.7 |
Black | 0.8 | |
Hispanic, Latine, or Spanish origin | 4.1 | |
Multiracial | 8.1 | |
White | 79.7 | |
Preferred not to respond | 1.6 | |
College Generation | Continuing gen | 87.0 |
First-gen | 12.2 | |
Does not know or preferred not to respond | 0.8 | |
Year in College | First | 71.5 |
Second | 20.3 | |
Third | 7.3 | |
Fourth | 0.8 | |
Major | Biological Sciences | 43.9 |
Other STEM Major | 40.7 | |
Non-STEM Major | 15.4 | |
Lecture Section | Lecturer A Section 1 | 32.5 |
Lecturer A Section 2 | 42.3 | |
Lecturer B | 17.9 | |
Lecturer C | 7.3 |
MSLQ.
As per MSLQ protocol, we calculated students’ IGO, EGO, and SE scores by averaging the responses for the items within each construct. Each student received a score for IGO, EGO, and SE for each survey.
Prior studies have provided evidence of validity for the MSLQ subscales in large undergraduate student populations (Credé and Phillips, 2011). For our study, we used confirmatory factor analysis to provide evidence of reasonable fit (CFI - 91.2, TLI - 89.6, RMSEA - 0.079), where reasonable fit is defined by CFI and TLI > 90.0 and RMSEA between 0.08 and 0.05 (Bentler, 1990; Fan et al., 1999). Cronbach's alpha internal consistency reliabilities for each construct were above (IGO α = 0.74, SE α = 0.92), or near (EGO α = 0.63) the accepted cutoff (α = 0.70) (Nunnally and Bernstein, 1994). In the original MSLQ study, EGO had a Cronbach's alpha of 0.62 (Pintrich et al., 1993), which aligned with our study. We ran a D'Agostino test for normality and found that the scores for each construct and grades were normally distributed (D'Agostino, 1971).
RQ 1: How do SE, IGO, and EGO change after assessment grades are returned in introductory biology?
We calculated descriptive statistics to describe the students’ SE, IGO, and EGO scores at each timepoint. Then we ran ANOVAs for each construct with Tukey post-hoc tests to analyze whether mean student scores for SE, IGO, and EGO were statistically different among the three timepoints.
RQ 2: How do the relationships between SE, motivation, and final grades change after assessment grades are returned in introductory biology?
We used structural equation modeling (SEM) to identify the direct and indirect links between SE, IGO, EGO, and final course grades at each survey timepoint (precourse, postassessment 1, and postassessment 2). SEM is a statistical method that examines hypothesized relationships between latent (e.g., constructs) and observed (e.g., grades) variables (Maruyama, 1998). We developed our model based on the relationships hypothesized by the integration of SE theory with goal orientation theory (Figure 1). This theory predicts that increased SE is related to positive increases in both IGO and EGO, and that an increase in each type of motivation can be related to greater student success in the form of higher final grades. In total, we constructed three SEMs (one for each data collection timepoint) to represent how the relationships between constructs and final course grades changed as students received assessment feedback throughout the semester.
Before we conducted SEM, we first scaled the scores for each student (IGO, EGO, and SE at each timepoint), as well as their final grade. Scores were scaled in terms of their SDs from the mean, making the values more easily comparable to one another. We used Akaike information criterion (AIC; Akaike, 1987) model selection to compare three sets of potential SEMs (Supplemental Table S1). The first potential models included relationships between SE, IGO, EGO, and final course grades without any random effects (e.g., course section or instructor); these had the best (lowest) AIC. The second and third SEM sets incorporated linear mixed effect models, testing how the addition of different candidate random effects impacted the relationships between the variables. In the second model, we added a random effect of class section (four levels) as a grouping variable. Similarly, in the third model, we added a random effect of instructor (three levels) as a grouping variable. We found that the models with the random effects had higher AIC (demonstrating lower model fit; Akaike, 1987) and the scores did not vary significantly between class section or lecturer. Therefore, we selected the first set of models to answer our research question. We conducted goodness-of-fit tests on our final models using each model's Fisher's C and chi-squared values to ensure that the models sufficiently captured the relationships within the data (Lefcheck, 2016).
All statistical tests were performed in R v. 4.3.1 (R Core Team, 2023) using the following packages: psych (Revelle, 2023), car (Fox and Weisberg, 2019), piecewiseSEM (Lefcheck, 2016), lme4 (Bates et al., 2015), and ggplot2 (Wickham, 2016).
Results
RQ 1: How do SE, IGO, and EGO Change After Assessment Grades are Returned in Introductory Biology?
We found that SE and IGO scores significantly decreased compared with precourse scores after students received grades from their first assessment. Precourse SE scores were significantly higher compared with postassessment 1 and postassessment 2 SE scores (p < 0.05; Figure 2; Table 5); there were no significant differences between postassessment 1 and postassessment 2 SE scores. Precourse IGO scores were significantly higher compared with postassessment 1 (p < 0.001) and postassessment 2 IGO scores (p < 0.05; Figure 3; Table 6); there were no significant differences between postassessment 1 and 2 IGO scores. We found no significant differences in students’ EGO scores at any of the timepoints (Figure 4; Table 7).
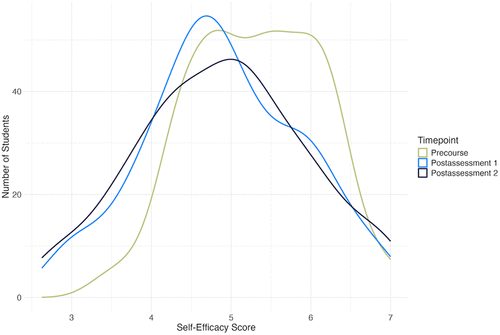
FIGURE 2. SE scores over the three timepoints surveyed showing the distribution of Likert scale averages for the 123 student respondents. 7 is the highest possible SE score. Precourse SE scores were significantly higher (p < 0.05) than postassessment 1 and postassessment 2 scores.
Mean | Median | Std. Dev. | Min. | Max. | |
---|---|---|---|---|---|
Precourse | 5.33 | 5.38 | 0.79 | 3.38 | 7 |
Postassessment 1 | 4.91 | 4.88 | 0.97 | 2.75 | 7 |
Postassessment 2 | 4.92 | 5.00 | 1.05 | 2.63 | 7 |
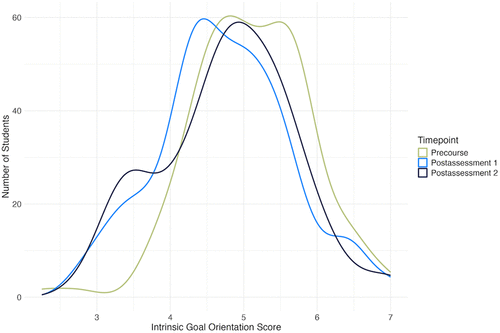
FIGURE 3. IGO scores over the three timepoints surveyed showing the distribution of Likert scale averages for the 123 student respondents. 7 is the highest possible IGO score. Precourse IGO scores were higher compared with postassessment 1 (p < 0.001) and postassessment 2 IGO scores (p < 0.05).
Mean | Median | Std. Dev. | Min. | Max. | |
---|---|---|---|---|---|
Precourse | 5.13 | 5.25 | 0.80 | 2.25 | 7 |
Postassessment 1 | 4.72 | 4.75 | 0.89 | 2.75 | 7 |
Postassessment 2 | 4.78 | 4.75 | 0.91 | 2.75 | 7 |
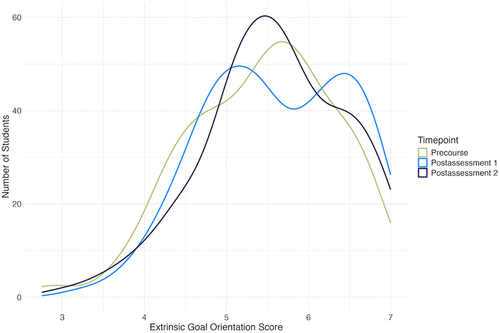
FIGURE 4. EGO scores over the three timepoints surveyed showing the distribution of Likert scale averages for the 123 student respondents. 7 is the highest possible EGO score. Mean EGO scores were not statistically different from one another.
Mean | Median | Std. Dev. | Min. | Max. | |
---|---|---|---|---|---|
Precourse | 5.45 | 5.50 | 0.88 | 2.75 | 7 |
Postassessment 1 | 5.59 | 5.50 | 0.87 | 3.25 | 7 |
Postassessment 2 | 5.58 | 5.50 | 0.86 | 3.00 | 7 |
RQ 2: How do the Relationships Between SE, Motivation, and Final Grades Change after Assessment Grades are Returned in Introductory Biology?
The three SEMs each had Fisher's C and chi-squared values above the p = 0.05 threshold (Table 8), indicating that the included paths sufficiently captured the variation in the data at each timepoint (Lefcheck, 2016).
Precourse Model | |||||
---|---|---|---|---|---|
Response | Predictor | Std. Estimate | Std. Error | DF | p Value |
EGO Score | SE Score | 0.4836 | 0.0879 | 121 | <0.001 |
IGO Score | SE Score | 0.6229 | 0.0765 | 121 | <0.001 |
Grades | SE Score | 0.1707 | 0.0767 | 119 | <0.05 |
Grades | EGO Score | −0.1059 | 0.0581 | 119 | 0.0709 |
Grades | IGO Score | −0.0981 | 0.0668 | 119 | 0.1446 |
Fisher's C = 2.486 (p = 0.288), Chi-Squared Test = 1.16 (p = 0.282), EGO R2 = 0.20, IGO R2 = 0.35, Grades R2 = 0.05 |
Postassessment 1 Model | |||||
---|---|---|---|---|---|
Response | Predictor | Std. Estimate | Std. Error | DF | p Value |
EGO Score | SE Score | 0.2828 | 0.0679 | 121 | <0.001 |
IGO Score | SE Score | 0.5770 | 0.0695 | 121 | <0.001 |
Grades | SE Score | 0.1852 | 0.0549 | 119 | <0.05 |
Grades | EGO Score | −0.0353 | 0.0559 | 119 | 0.5281 |
Grades | IGO Score | −0.0895 | 0.0549 | 119 | 0.1035 |
Fisher's C = 0.809 (p = 0.667), Chi-Squared Test = 0.19 (p = 0.663), EGO R2 = 0.13, IGO R2 = 0.36, Grades R2 = 0.09 |
Postassessment 2 Model | |||||
---|---|---|---|---|---|
Response | Predictor | Std. Estimate | Std. Error | DF | p Value |
EGO Score | SE Score | 0.2633 | 0.0622 | 121 | <0.001 |
IGO Score | SE Score | 0.4648 | 0.0695 | 121 | <0.001 |
Grades | SE Score | 0.2315 | 0.0446 | 119 | <0.001 |
Grades | EGO Score | −0.0471 | 0.0531 | 119 | 0.3768 |
Grades | IGO Score | −0.0871 | 0.0475 | 119 | 0.0691 |
Fisher's C = 0.157 (p = 0.925), Chi-Squared Test = 0.009 (p = 0.923), EGO R2 = 0.13, IGO R2 = 0.27, Grades R2 = 0.19 |
There were significant relationships between constructs that were consistent across each of the three timepoints (Figure 5). Our expectation, based on theory, was that SE, IGO, and EGO would be related and that both IGO and EGO would mediate the positive relationship between SE and final course grades. Across all three timepoints, we found evidence that SE scores were positively related to EGO scores (precourse: β = 0.4836 ± 0.0879, p < 0.001; postassessment 1: β = 0.2828 ± 0.0679, p < 0.001; postassessment 2: β = 0.2633 ± 0.0622, p < 0.001) and IGO scores (precourse: β = 0.6229 ± 0.0765, p < 0.001; postassessment 1: β = 0.5770 ± 0.0695, p < 0.001; postassessment 2: β = 0.4648 ± 0.0695, p < 0.001). However, we did not find a significant relationship between EGO and/or IGO scores, and final course grades in any of the models (Table 8), indicating that neither IGO nor EGO mediated the relationship between student SE and final grades at any timepoint.
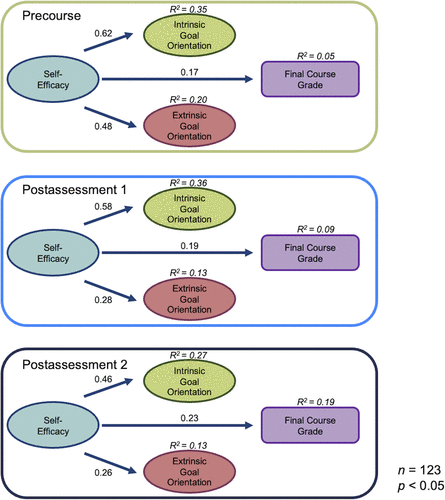
FIGURE 5. Path diagrams for each of the structural equation models. The precourse model is noted in the green box (top), the postassessment 1 model is noted in blue (middle), and the postassessment 2 model is noted in navy blue (bottom). Numbers above arrows are standardized beta coefficients (e.g., a one unit increase in precourse SE results in a 0.27 unit increase in final course grades). R2 values are noted above each variable and indicate the amount of variation explained by the model (e.g., the precourse model explains 5% of the variation in final course grades).
There was a positive direct effect of SE scores on final course grades in each of the three models (precourse: β = 0.1707 ± 0.0767, p < 0.05; postassessment 1: β = 0.1852 ± 0.0549, p < 0.05; postassessment 2: β = 0.2315 ± 0.0446, p < 0.001). Students’ SE scores were therefore the primary predictor of final course grades at all survey timepoints. This relationship strengthened over time, with the amount of variation in final course grades explained by SE scores increasing from 5% at the precourse time point to 9% postassessment 1, and 19% postassessment 2. This indicates that over the semester the strength of the relationship between SE level and final grades increased.
DISCUSSION
This study explored student measures of SE, IGO, and EGO before an introductory biology course started and after students had received their first two summative assessment grades in four introductory biology classes. We also examined the relationships among SE, IGO, EGO levels, and final grades at these three timepoints. After the first assessment grades were posted, SE and IGO scores were lower compared with the start of the semester, while EGO scores remained stable. While we cannot directly link changes in SE and IGO scores to the receipt of assessment grades, our study adds new information to the literature about key moments when students received this important feedback, which theory suggests may be used to inform SE levels via enactive mastery experiences (Bandura, 1986). This finding is important given our second main finding that there was a strong relationship between SE level and final grades that became stronger over time. Importantly, this finding did not differ among course sections, despite different assessment structures. Thus, this study supports and builds upon many of the tenets of SE theory by demonstrating that increasing SE levels are related to more positive outcomes on final grades and higher motivation. These results can be used by instructors of these courses to think about how and when to find ways to support student SE, and therefore success, in introductory biology.
Finding 1: SE and IGO Scores Decreased after Assessment 1 Grades were Returned Compared with Precourse Levels
Our findings revealed that both SE and one type of motivation, IGO, were lower after the first assessment grades were returned to students compared with precourse levels. This aligns with other studies that found decreases in factors such as SE, motivation, and self-regulation skills over time for students (Wigfield et al., 1996; Pintrich, 2000b; Zusho et al., 2003; Young et al., 2018) but contradicts findings of increasing SE over time in other introductory biology courses (Ainscough et al., 2016; Cole and Beck, 2022). These differences may reflect the timing of when the constructs were measured or the context of these courses at our institution. The decrease in SE we found was relative to a precourse baseline measure, which was not taken in the other studies on introductory biology courses (Ainscough et al., 2016; Cole and Beck, 2022). For many of the students in the sample, that baseline measure was prior to starting their first college biology course as a freshman, suggesting that their level of SE was informed by academic experiences prior to college. Given that many students entering our institution did well in high school, this may have contributed to them having higher confidence before starting the course than if a baseline measure was taken even 1 or 2 weeks after it started. We do not know when the decline in SE and IGO occurred, or whether it was related to their assessment results, but many of the students in our sample received their first assessment feedback about 2 weeks after the class started, suggesting SE and IGO decline quickly. Therefore, our study adds new information on the timing of potential shifts in SE and IGO and suggests the need to probe SE and IGO shifts at a finer temporal scale and examine whether assessment feedback is a factor in these shifts.
Whether assessment feedback is a factor in SE and IGO shifts or not, our results suggest that supporting student SE after the first assessment grades are returned in these courses could be a critical timepoint for intervention. Instructors, for example, may support student SE through verbal persuasion or bolstering student emotional or affective states (Bandura, 1977; 1997) or through the use of positive noncontent instructor talk (Seidel et al., 2015). Bolstering student motivation through a positive class climate or making the goals of the course salient may also help to increase SE given the correlations between the two (Ames and Archer, 1988; Ames, 1992). Given that student perceptions of their performance can be influenced by instructor feedback and support (Chan and Lam, 2010; Beattie et al., 2016), consciously finding ways to support recovery of SE in these courses might be important for increasing student success. Uncovering exactly which factors influenced the decreases in SE and IGO is outside the scope of this study, but we suggest that future research could use qualitative methods to understand more about how students perceive the climate in a course and how that relates to their SE and motivation.
Assessment is one factor instructors have control over that is likely to influence student SE levels (Gibbs and Simpson, 2005; Schinske and Tanner, 2014). For instance, formative assessments are typically lower stakes opportunities to receive feedback and help students learn; while summative assessments usually provide students feedback in the form of grades and measures of learning, although they are also considered opportunities to learn as well (Cooper, 2000; Colbran et al., 2016; Bin Mubayrik, 2020). In one study, researchers found that students who were given only formative assessments over 15 class sessions had higher overall class performance, motivation, and self-regulated learning skills than those who were given only summative assessments (Ismail et al., 2022). The instructor administering formative assessments in that study provided constructive feedback to students to help them learn and progress, while the instructor administering summative assessments limited their feedback to yes/no, true/false, and grades on the midterm and final (Ismail et al., 2022). Thus, both assessment structure and the amount of student feedback may interact to support learning and promote positive SE.
The instructors in our study had different assessment structures, however, and we did not find evidence that these differences were related to students’ SE, IGO, EGO or final course grades, or the relationships among them. Lecturer A gave smaller point value quizzes more often compared with the other Lecturers in order to try to ease student exam anxiety and help them succeed. It is possible that the benefit of lower point values may have been offset by the pressure of having a quiz every 2 weeks; however, this would need to be explored as a separate study. Lecturers B and C had slightly more points allocated for formative assessment activities such as in class clickers, worksheets, and quizzes. Clearly, assessment in introductory biology introduces myriad factors that could be explored including assessment structure, exam wrappers, dropped grades, etc. and it is unknown whether courses that differed more in these assessment factors than ours did would have resulted in differences among course sections or instructors. We also did not collect individual assessment grades at each timepoint to be able to model whether the assessment grade itself was a factor in SE and motivation level changes. A study designed to probe these questions may provide empirical data to instructors to create assessment structures that can support high SE and should be a focus of future investigations.
Finding 2: SE was Positively Related to Student Motivation and Final Grades in Introductory Biology
Based on SE theory, we expected to see that IGO and EGO may mediate the relationship between SE and final grades. Although we did find that SE had a positive relationship with both IGO and EGO, we did not find evidence that IGO and EGO mediated the relationship between SE and final course grade. This was surprising because previous studies have found a direct effect of both IGO and EGO on final course grades (Wolters et al., 1996; Middleton and Midgley 1997; Pajares et al., 2000; Mattern, 2005). However, research does suggest that there are differences between two types of EGO: approach and avoidance (Elliot and Harackiewicz, 1996; Church et al., 2001). This differentiation between the two was not accounted for in our study and may be one reason we do not see an effect of EGO on final course grade. While our results suggest that motivation is not related to final course grades, this does not necessarily mean that motivation does not drive student success. It may be that final course grades are not the sole indicator of success, particularly for students who do not define success in introductory biology as related to performance (Von der Mehden et al., 2024). As such, future work should investigate the role motivation plays in student success when success is not based on performance outcomes (e.g., an ungraded course).
Students with higher SE scores tended to have higher final course grades, which aligns with previous research suggesting that SE has a positive relationship with course grades (Chemers et al., 2001; Britner and Pajares, 2006; Hsieh et al., 2007). We found that SE scores also became stronger predictors of final grades as the course progressed, which is a novel contribution to the literature on SE and introductory biology courses. One possibility for our courses is that receiving a grade gives students the opportunity to make adjustments to improve their performance. Subsequent grades serve as additional feedback, helping them evaluate whether their adjustments were successful (Chan and Lam, 2010; Beattie et al., 2016). This metacognitive process can be amplified by guidance from instructors, helping students decide which adjustments to make to their study strategies to become more successful (Stanton et al., 2019). However, SE is a construct that is likely influenced by many factors and more studies need to be done in introductory biology to understand how to enhance it in these courses.
Limitations and Future Work
Although the design of this study was to measure SE, IGO, and EGO as students were receiving assessment grades, the results cannot be directly attributed to receiving feedback on assessments. The timepoints we selected for surveying were immediately after students received their grades on assessments, but it is possible that students’ SE and motivation were changing as a result of other factors (e.g., course structure, sociodemographic characteristics, other courses, etc.). Interviewing students about what specific factors impacted their SE would be a direct way to assess this in a future study. In addition, future research could focus on measuring SE changes on a finer scale to explore exactly when SE decreases for students. One way to do this is by employing the experience sampling method which can measure a construct more often by sending text messages to students on a more frequent basis (Weatherton et al., 2024).
This study was carried out at one research-intensive institution in the southeastern United States; therefore, these results are not generalizable to other student populations. It was also specific to the context of large introductory majors’ biology courses, and in this case, one that was populated by many first-year students taking the first course for their biology degree. Assessment and feedback may look very different for students in smaller classes, or classes not required as part of their major. In addition, the participants for this study shared largely homogenous demographics and do not represent many undergraduate students. Given that previous studies have established that various assessment structures differentially affect students belonging to different demographic groups (Ballen et al., 2017; Cotner and Ballen, 2017; Salehi et al., 2019), future studies should explore more diverse populations.
CONCLUSION
This study investigated how relationships between SE, motivation, and final course grades changed over three critical timepoints in an introductory biology course. SE and IGO scores decreased among the student participants after the first assessment grades were provided, while EGO scores were unchanged. We found that the strength of the relationship between SE and final course grades increased over time, emphasizing its crucial role in academic success. Contrary to what was predicted by theory, IGO and EGO did not mediate the relationship between SE and grades, suggesting that motivation did not directly impact final academic performance. These findings highlight the need for educational strategies that support students’ SE development such as a positive classroom climate and assessment structures and feedback that support SE. This study also suggests that the time period after students receive their first exam grades may be a critical time for strategies to boost SE.
ACKNOWLEDGMENTS
The authors thank the students and faculty who participated in this study. We thank the members of the Gaoue Lab and the Schussler Lab for continuous feedback and support during data collection and manuscript preparation stages. We also thank the editors and reviewers for their thoughtful feedback on this manuscript.
REFERENCES
- 1987). Factor analysis and AIC. Psychometrika, 52, 317–332. Google Scholar (
- 2016). Changes in biology self-efficacy during a first-year university course. CBE—Life Sciences Education, 15(2), ar19. https://doi.org/10.1187/cbe.15-04-0092 Link, Google Scholar (
- 1992). Classrooms: Goals, structures, and student motivation. Journal of Educational Psychology, 84(3), 261–271. https://doi.org/10.1037/0022-0663.84.3.261 Google Scholar (
- 1988). Achievement goals in the classroom: Students’ learning strategies and motivation processes. Journal of Educational Psychology, 80(3), 260–267. Google Scholar (
- 1998). Self-efficacy as a predictor of academic performance in science. Journal of Advanced Nursing, 27(3), 596–603. https://doi.org/10.1046/j.1365-2648.1998.00550.x Medline, Google Scholar (
- 2017). Exams disadvantage women in introductory biology. PLoS ONE, 12(10), e0186419. https://doi.org/10.1371/journal.pone.0186419 Medline, Google Scholar (
- 1977). Self-efficacy: Toward a unifying theory of behavioral change. Advances in Behaviour Research and Therapy, 1(4), 139–161. https://doi.org/10.1016/0146-6402(78)90002-4 Google Scholar (
- 1986). Social foundations of thought and action: A social cognitive theory, 617. pp. xiii. Englewood Cliffs, NJ: Prentice Hall. Google Scholar (
- 1997). Self-efficacy: The Exercise of Control (pp. ix, 604). New York: W H Freeman/Times Books/Henry Holt & Co. Google Scholar (
- 2018). The impact of mastery feedback on undergraduate students’ self-efficacy beliefs. Studies in Educational Evaluation, 59, 58–66. https://doi.org/10.1016/j.stueduc.2018.03.002 Google Scholar (
- 2015). Fitting Linear Mixed-Effects Models Using lme4. Journal of Statistical Software, 67(1), 148. https://doi.org/10.18637/jss.v067.i01 Google Scholar (
- 2016). The role of performance feedback on the self-efficacy–performance relationship. Sport, Exercise, and Performance Psychology, 5(1), 1–13. https://doi.org/10.1037/spy0000051 Google Scholar (
- 1990). Comparative fit indexes in structural models. Psychological Bulletin, 107(2), 238–246. https://doi.org/10.1037/0033-2909.107.2.238 Medline, Google Scholar (
- 2020). New trends in formative-summative evaluations for adult education. Sage Open, 10(3). https://doi.org/10.1177/2158244020941006 Google Scholar (
- 2006). Sources of science self-efficacy beliefs of middle school students. Journal of Research in Science Teaching, 43(5), 485–499. https://doi.org/10.1002/tea.20131 Google Scholar (
- 2007). Clickers in the large classroom: Current research and best-practice tips. CBE—Life Sciences Education, 6(1), 9–20. https://doi.org/10.1187/cbe.06-12-0205 Link, Google Scholar (
- 2010). Effects of different evaluative feedback on students’ self-efficacy in learning. Instructional Science, 38(1), 37–58. https://doi.org/10.1007/s11251-008-9077-2 Google Scholar (
- 2001). Academic self-efficacy and first year college student performance and adjustment. Journal of Educational Psychology, 93(1), 55–64. https://doi.org/10.1037/0022-0663.93.1.55 Google Scholar (
- 2001). Perceptions of classroom environment, achievement goals, and achievement outcomes. Journal of Educational Psychology, 93(1), 43–54. https://doi.org/10.1037/0022-0663.93.1.43 Google Scholar (
- 2016). Animation and multiple-choice questions as a formative feedback tool for legal education. The Law Teacher, 51(3), 249–273. https://doi.org/10.1080/03069400.2016.1162077 Google Scholar (
- 2022). Developmental trajectories of student self-perception over a yearlong introductory biology sequence. CBE—Life Sciences Education, 21(3), ar59. https://doi.org/10.1187/cbe.21-12-0326 Medline, Google Scholar (
- 2000). Facilitating learning from formative feedback in level 3 assessment. Assessment & Evaluation in Higher Education, 25(3), 279–291. https://doi.org/10.1080/713611435 Google Scholar (
- 2020). Trajectories of motivation and their academic correlates over the first year of college. Contemporary Educational Psychology, 63, 101907. https://doi.org/10.1016/j.cedpsych.2020.101907 Google Scholar (
- 2017). Can mixed assessment methods make biology classes more equitable? PLoS ONE, 12(12), e0189610. https://doi.org/10.1371/journal.pone.0189610 Medline, Google Scholar (
- 2011). A meta-analytic review of the motivated strategies for learning questionnaire. Learning and Individual Differences, 21(4), 337–346. https://doi.org/10.1016/j.lindif.2011.03.002 Google Scholar (
- 1971). An omnibus test of normality for moderate and large size samples. Biometrika, 58(2), 341. Google Scholar (
- 2011). Self-efficacy, goal orientations and learning strategies as mediators between preceding and subsequent academic achievement. Learning and Individual Differences, 21(2), 191–195. https://doi.org/10.1016/j.lindif.2011.01.003 Google Scholar (
- 1988). A social-cognitive approach to motivation and personality. Psychological Review, 95(2), 256–273. Google Scholar (
- 1996). Approach and avoidance achievement goals and intrinsic motivation: A mediational analysis. Journal of Personality and Social Psychology, 70(3), 461–475. https://doi.org/10.1037/0022-3514.70.3.461 Google Scholar (
- 2001). A 2 × 2 achievement goal framework. Journal of Personality and Social Psychology, 80(3), 501–519. https://doi.org/10.1037/0022-3514.80.3.501 Medline, Google Scholar (
- 1999). Effects of sample size, estimation methods, and model specification on structural equation modeling fit indexes. Structural Equation Modeling: A Multidisciplinary Journal, 6, 56–83. https://doi.org/10.1080/10705519909540119 Google Scholar (
- 2019). An R Companion to Applied Regression, 3rd ed Thousand Oaks, CA: Sage. Retrieved from https://socialsciences.mcmaster.ca/jfox/Books/Companion/ Google Scholar (
- 2016). Changing learning behaviour: Self-efficacy and goal orientation in PBL groups in higher education. International Journal of Educational Research, 75, 146–158. https://doi.org/10.1016/j.ijer.2015.11.001 Google Scholar (
- 2005). Conditions under which assessment supports students’ learning. Learning and Teaching in Higher Education, 3–31. Google Scholar (
- 1997). Predictors and consequences of achievement goals in the college classroom: Maintaining interest and making the grade. Journal of Personality and Social Psychology, 73(6), 1284–1295. https://doi.org/10.1037/0022-3514.73.6.1284 Google Scholar (
- 2000). Short-term and long-term consequences of achievement goals: Predicting interest and performance over time. Journal of Educational Psychology, 92(2), 316–330. https://doi.org/10.1037/0022-0663.92.2.316 Google Scholar (
- 2007). A closer look at college students: Self-efficacy and goal orientation. Journal of Advanced Academics, 18(3), 454–476. https://doi.org/10.4219/jaa-2007-500 Google Scholar (
- 2022). Formative vs. summative assessment: Impacts on academic motivation, attitude toward learning, test anxiety, and self-regulation skill. Language Testing in Asia, 12(1), 40. https://doi.org/10.1186/s40468-022-00191-4 Google Scholar (
- 2018). Peer vs. self-grading of practice exams: Which is better? CBE—Life Sciences Education, 17(3), es44. https://doi.org/10.1187/cbe.18-04-0052 Link, Google Scholar (
- 2013). Self-efficacy and academic achievement: Why do implicit beliefs, goals, and effort regulation matter? Learning and Individual Differences, 25, 67–72. https://doi.org/10.1016/j.lindif.2013.01.005 Google Scholar (
- 2016). piecewiseSEM: Piecewise structural equation modeling in R for ecology, evolution, and systematics. Methods in Ecology and Evolution, 7(5), 573–579. 10.1111/2041-210X.12512 <https://doi.org/10.1111/2041-210X.12512>. Google Scholar (
- 1986). Self-efficacy in the prediction of academic performance and perceived career options. Journal of Counseling Psychology, 33(3), 265–269. https://doi.org/10.1037/0022-0167.33.3.265 Google Scholar (
- 1998). Basics of structural equation modeling. Sage Publications, Inc. https://doi.org/10.4135/9781483345109 Google Scholar (
- 2005). College students’ goal orientations and achievement. International Journal of Teaching and Learning in Higher Education, 17(1), 27–32. Google Scholar (
- 1997). Avoiding the demonstration of lack of ability: An under-explored aspect of goal theory. Journal of Educational Psychology, 89(4), 710–718. Google Scholar (
- 2004). From vulnerability to resilience: Learning orientations buffer contingent self-esteem from failure. Psychological Science, 15(12), 801–805. Medline, Google Scholar (
- 1994). Psychometric Theory, 3rd ed. New York: McGraw-Hill. Google Scholar (
- 2013). Believe, and you will achieve: Changes over time in self-efficacy, engagement, and performance. Applied Psychology: Health and Well-Being, 5(2), 225–247. https://doi.org/10.1111/aphw.12008 Medline, Google Scholar (
- 1996). Self-efficacy beliefs in academic settings. Review of Educational Research, 66(4), 543–578. https://doi.org/10.2307/1170653 Google Scholar (
- 2000). Relation between achievement goals and self-beliefs of middle school students in writing and science. Contemporary Educational Psychology, 25(4), 406–422. https://doi.org/10.1006/ceps.1999.1027 Medline, Google Scholar (
- 2000a). Multiple goals, multiple pathways: The role of goal orientation in learning and achievement. Journal of Educational Psychology, 92(3), 544–555. https://doi.org/10.1037/0022-0663.92.3.544 Google Scholar (
- 2000b). The role of goal orientation in self-regulated learning. Handbook of Self-Regulation, 451–529. Cambridge, MA: Academic. Google Scholar (
- 1990). Motivational and self-regulated learning components of classroom academic performance. Journal of Educational Psychology, 82(1), 33–40. https://doi.org/10.1037/0022-0663.82.1.33 Google Scholar (
- 1991). A manual for the use of the Motivated Strategies for Learning Questionnaire (MSLQ), National Center for Research to Improve Postsecondary Teaching and Learning, 1–76. Ann Arbor, MI. Retrieved June 2024, from https://eric.ed.gov/?id=ED338122 Google Scholar (
- 1993). Reliability and predictive validity of the Motivated Strategies for Learning Questionnaire (Mslq), Retrieved March 2024, from https://journals.sagepub.com/doi/abs/10.1177/0013164493053003024 Google Scholar (
R Core, Team . (2023). R: A language and environment for statistical computing. R Foundation for Statistical Computing, Vienna, Austria. Retrieved March, 7 2023, from https://www.R-project.org/ Google Scholar- 2023). psych: Procedures for psychological,psychometric, and personality research. Northwestern University, Evanston, Illinois. R package version 2.3.9. Retrieved May 2024, from <https://CRAN.R-project.org/package=psych>. Google Scholar (
- 2012). lavaan: An R package for structural equation modeling. Journal of Statistical Software, 48(2), 1–36. https://doi.org/10.18637/jss.v048.i02 Google Scholar (
- 2019). Gender performance gaps across different assessment methods and the underlying mechanisms: The case of incoming preparation and test anxiety. Frontiers in Education, 4, 107. https://doi.org/10.3389/feduc.2019.00107 Google Scholar (
- 2014). Teaching more by grading less (or differently). CBE—Life Sciences Education, 13(2), 159–166. https://doi.org/10.1187/cbe.cbe-14-03-0054 Link, Google Scholar (
- 1984). Self-efficacy perspective on achievement behavior. Educational Psychologist, 19(1), 48–58. https://doi.org/10.1080/00461528409529281 Google Scholar (
- 2015). Beyond the biology: A systematic investigation of noncontent instructor talk in an introductory biology course. CBE—Life Sciences Education, 14(4), ar43. https://doi.org/10.1187/cbe.15-03-0049 Link, Google Scholar (
- 2019). Knowledge of learning makes a difference: A comparison of metacognition in introductory and senior-level biology students. CBE—Life Sciences Education, 18(2), ar24. https://doi.org/10.1187/cbe.18-12-0239 Link, Google Scholar (
- 2006). Sources of academic and self-regulatory efficacy beliefs of entering middle school students. Contemporary Education Psychology, 31, 125–141. http://dx.doi.org/10.1016/j.cedpsych.2005.03.002 Google Scholar (
- 2011). Factors affecting students’ self-efficacy in higher education. Educational Research Review, 6(2), 95–108. https://doi.org/10.1016/j.edurev.2010.10.003 Google Scholar (
- 2024). Student perspectives of success and failure in biology lecture: Multifaceted definitions and misalignments. CBE—Life Sciences Education, 23(3), ar29. https://doi.org/10.1187/cbe.23-12-0243 Medline, Google Scholar (
- 2024). Beyond pre-post surveys: Exploring validity evidence for the use of experience sampling methods to measure student anxiety in introductory biology. bioRxiv. https://doi.org/10.1101/2024.06.06.597780 Google Scholar (
- 2016). ggplot2: Elegant graphics for data analysis. New York: Springer-Verlag. Google Scholar (
- 1996). Development between the ages of ll and 25. Handbook of Educational Psychology, 148–185. Macmillan Library Reference USA: Prentice Hall International. Google Scholar (
- 1996). The relation between goal orientation and students’ motivational beliefs and self-regulated learning. Learning and Individual Differences, 8(3), 211–238. https://doi.org/10.1016/S1041-6080(96)90015-1 Google Scholar (
- 2018). Motivational decline and recovery in higher education STEM courses. International Journal of Science Education, 40(9), 1016–1033. https://doi.org/10.1080/09500693.2018.1460773 Google Scholar (
- 1992). Self-motivation for academic attainment: The role of self-efficacy beliefs and personal goal setting. American Educational Research Journal, 29(3), 663–676. https://doi.org/10.2307/1163261 Google Scholar (
- 2003). Skill and will: The role of motivation and cognition in the learning of college chemistry. International Journal of Science Education, 25(9), 1081–1094. https://doi.org/10.1080/0950069032000052207 Google Scholar (